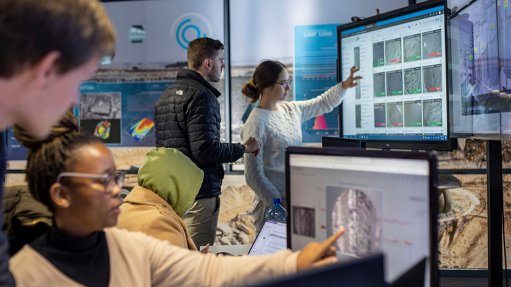
DETECT AND EXTRACT
The particle size analyser can be used to prevent the truck from tipping, in the event of oversize material, or to examine the mining performance
Photo by: Stone Three
The iron-ore mining sector continues to develop and enhance its productivity by introducing various types of sensors and information-capturing devices throughout the value chain to reduce production time and ensure fewer project interruptions. The devices achieve these goals using artificial intelligence (AI) sequencing.
Fourth Industrial Revolution mining solutions provider Stone Three process data analyst Bernard Coetzee says combining multiple data streams with several inputs and outputs is a very intricate task, even more so when there is complex interaction between the inputs and outputs, which cannot be easily defined.
“This is why AI is significant in the devices for iron-ore mining – it enables miners to decipher huge quantities of information, quickly and accurately,” he enthuses.
Further, he notes downtime, owing to run-of-mine (RoM) material that is out of specification, is a large contributor to overall plant downtime and, thus, also to plant availability.
“It is, therefore, vital to not only ensure a constant and stable RoM particle size distribution but also prevent any oversize or foreign material from entering the process.”
At an iron-ore mine in South Africa, Stone Three’s truck camera particle size analyser is used to achieve both these goals. It examines images taken as, or before, the truck tips. It then uses the machine learning (ML) models to examine the particles present, and finally presents an observed reading of the material.
The impact of this information is far-reaching, with some use cases including tracking the percentage fines and how that relates to mining and dense- media separation performance, and real-time oversize particle detection and prevention, says Coetzee.
The particle size analyser can be used to prevent the truck from tipping, in the event of oversize material, or to examine the mining performance by building longer-term analysing trends. It can also detect foreign objects during iron-ore mining and this information can be used to alert and prevent the trucks from tipping and introducing the foreign object into the process.
The analyser detects these interruptions using its trained ML models, which examine the camera observing the tipping bins. It also allows for longer-term investigations into the mining performance.
Coetzee adds that there are two main challenges with ML models.
Firstly, since it uses a visual input with the cameras, it can examine only what can be seen and, therefore, is unable to detect the material on the truck bed and make inferences.
Secondly, the models are only trained on previous production events; thus, a sufficient history of similar events is required to train the model and improve its accuracy.
He comments that Stone Three’s process monitoring and diagnostic services are designed to complement the on-site monitoring services through trained and experienced process engineers and subject matter experts, who monitor client-specific key performance indicators and investigate possible causes.
“With the Stone Three process monitoring, and diagnostics team and site workers working together, the time from when issues are identified to when the issue is resolved is reduced considerably,” Coetzee concludes.